Have you ever wondered how artificial intelligence (AI) is transforming the stock market landscape? If you are interested in investing in stocks, you might want to know how AI can help you make better trading decisions, reduce risks, and increase returns. In this article, we will provide a comprehensive overview of AI-powered stock trading, including its impact on traders, regulators, consumers, and society at large.
AI is a branch of computer science that aims to create machines and systems that can perform tasks that normally require human intelligence, such as learning, reasoning, problem-solving, and decision-making. AI can be applied to various domains and industries, such as healthcare, education, entertainment, and finance. In particular, AI has been widely used in stock trading, which is the activity of buying and selling stocks or shares of companies on the stock market.
Stock trading can be challenging and complex, as it involves analyzing large amounts of data, predicting market movements, executing trades at the right time, and managing risks. Traditionally, stock traders rely on their own knowledge, experience, intuition, and emotions to make trading decisions. However, human traders have some limitations, such as cognitive biases, information overload, emotional stress, and slow reaction time. These limitations can affect the accuracy and efficiency of their trading performance.
This is where AI comes in. AI-powered stock trading refers to the use of artificial intelligence, predictive analytics, and machine learning to analyze historical market and stock data, get investment ideas, build portfolios, and automatically buy and sell stocks. AI-powered stock trading can offer several benefits to traders, such as:
- Speed: AI can process huge amounts of data faster than humans and execute trades in milliseconds or microseconds.
- Accuracy: AI can eliminate human errors and biases and provide more reliable and consistent results.
- Efficiency: AI can optimize trading strategies and outcomes by finding the best opportunities and prices in the market.
- Scalability: AI can handle multiple trades and portfolios simultaneously and adapt to changing market conditions.
- Profitability: AI can enhance trading performance and generate higher returns for traders.
However, AI-powered stock trading also poses some challenges, such as:
- Transparency: AI algorithms can be complex and opaque, making it difficult to understand how they work and why they make certain decisions.
- Accountability: AI algorithms can make mistakes or cause harm, raising questions about who is responsible for their actions and outcomes.
- Privacy: AI algorithms can collect and use personal or sensitive data from traders or consumers, raising concerns about data protection and security.
- Ethics: AI algorithms can have social or environmental impacts, raising issues about fairness, justice, and sustainability.
In this article, we will explore these benefits and challenges in more detail. We will also discuss some of the types, tools, and techniques of AI-powered stock trading. We will provide some examples of companies that are using or developing AI for stock trading. Finally, we will look into some of the trends and predictions for the future of AI in the stock market.
The main research questions that we will address in this article are:
- How does AI-powered stock trading work?
- What are the advantages and disadvantages of AI-powered stock trading?
- What are some of the examples of AI-powered stock trading applications?
- What are some of the ethical considerations surrounding AI-powered stock trading?
- What are some of the future developments that traders should watch for?
How AI-Powered Stock Trading Bots Are Changing the Game for Retail Investors
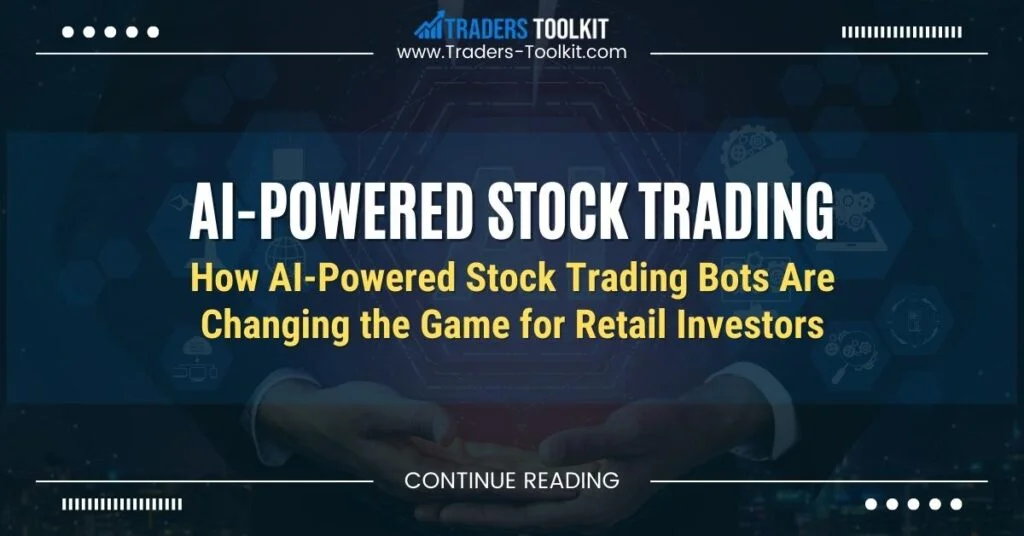
One of the most common applications of AI-powered stock trading is trading bots. Trading bots are software programs that use predefined rules or algorithms to automatically buy and sell stocks on behalf of traders. Trading bots can operate 24/7 without human intervention or supervision. They can also execute trades faster and more efficiently than human traders.
Trading bots are not a new phenomenon in the stock market. They have been used by institutional investors, such as hedge funds or banks, for decades. However, with the advancement of technology and the availability of data, trading bots have become more accessible and affordable for retail investors, who are individual investors who trade stocks for their own personal accounts.
There are several benefits of using AI-powered trading bots for retail investors:
- Accessibility: Retail investors can access various online platforms or apps that offer AI-powered trading bots. They do not need to have advanced technical skills or knowledge to use them. They can simply choose a bot that suits their preferences or goals, set up their account, and start trading.
- Affordability: Retail investors do not need to pay high fees or commissions to use AI-powered trading bots. They can also save money by avoiding human errors or emotional mistakes that can lead to losses.
- Diversity: Retail investors can choose from a wide range of AI-powered trading bots that have different features, functions, and strategies. They can also customize their bots to fit their own risk appetite, trading style, and budget.
- Performance: Retail investors can improve their trading performance and results by using AI-powered trading bots. They can take advantage of the speed, accuracy, and efficiency of AI to find the best opportunities and prices in the market. They can also leverage the predictive power and analytical capabilities of AI to forecast market movements and make informed decisions.
Some examples of popular and successful AI-powered trading bots in the market are:
- Trade Ideas: Trade Ideas is an AI-powered stock scanner that helps traders find profitable trading opportunities. It uses machine learning to analyze millions of data points and generate trade signals based on various criteria, such as price, volume, trend, pattern, etc. It also provides backtesting and simulation tools to help traders test and optimize their strategies.
- Alpaca: Alpaca is an AI-powered commission-free stock trading platform that allows traders to create and run their own trading bots. It uses natural language processing to understand traders’ commands and execute trades accordingly. It also provides a cloud-based API that enables traders to connect their bots to various data sources, brokers, and exchanges.
- Stock Master: Stock Master is an AI-powered stock trading app that helps traders manage their portfolios and execute trades. It uses deep learning to analyze market data and provide real-time insights, recommendations, and alerts. It also provides a social trading feature that allows traders to follow and copy other successful traders.
AI-powered trading bots are changing the game for retail investors by making stock trading more accessible, affordable, diverse, and profitable. They are leveling the playing field for retail investors who want to compete with institutional investors in the stock market.
Predictive Power: Using AI for Stock Price Forecasting and Analysis
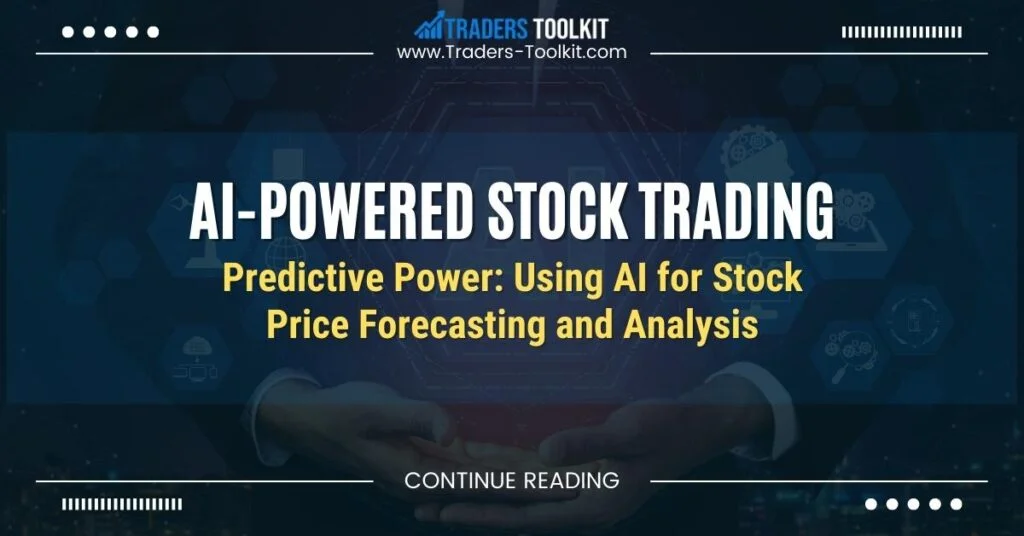
Another important application of AI-powered stock trading is stock price forecasting and analysis. Stock price forecasting and analysis is the process of predicting the future price movements of stocks based on historical data, current trends, and other factors. Stock price forecasting and analysis are crucial for traders who want to make profitable trading decisions.
Traditionally, stock price forecasting and analysis rely on two main methods: fundamental analysis and technical analysis. Fundamental analysis is the method of evaluating the intrinsic value of a stock based on its financial performance, growth potential, competitive advantage, etc. Technical analysis is the method of studying the price patterns, trends, indicators, etc., of a stock using statistical tools and charts.
However, both methods have some limitations, such as:
- Data limitations: Fundamental analysis and technical analysis require a lot of data to be accurate and reliable. However, not all data is available or accessible for all stocks or markets. Some data may also be outdated, incomplete, or inaccurate.
- Human limitations: Fundamental analysis and technical analysis involve a lot of human judgment and interpretation. However, humans can be prone to errors, biases, or emotions that can affect their analysis and predictions.
- Complexity limitations: Fundamental analysis and technical analysis can be complex and time-consuming. They can also be difficult to apply to volatile or unpredictable markets or situations.
This is where AI comes in. AI-powered stock price forecasting and analysis uses machine learning algorithms to analyze large amounts of data, identify patterns, learn from past experiences, and make predictions based on various factors. AI-powered stock price forecasting and analysis can offer several advantages over traditional methods, such as:
- Data advantages: AI can process huge amounts of data faster and more efficiently than humans. It can also access and use different types of data sources, such as news articles, social media posts, online reviews, etc., that may not be considered by traditional methods.
- Human advantages: AI can eliminate human errors and biases and provide more objective and consistent results. It can also adapt to changing market conditions and learn from new data or feedback.
- Complexity advantages: AI can handle complex and nonlinear relationships between variables that may not be captured by traditional methods. It can also provide more accurate and precise predictions for short-term or long-term horizons.
Some examples of AI models and techniques used for stock price forecasting and analysis are:
- Neural networks: Neural networks are a type of machine learning algorithm that mimics the structure and function of the human brain. They consist of layers of interconnected nodes that process information and learn from data. Neural networks can be used for stock price forecasting and analysis by learning from historical price data and generating predictions based on various inputs.
- Deep learning: Deep learning is a branch of machine learning that uses multiple layers of neural networks to learn from complex or high-dimensional data. Deep learning can be used for stock price forecasting and analysis by extracting features from raw data, such as images, text, or audio, and using them to make predictions based on various factors.
- Natural language processing: Natural language processing (NLP) is a branch of artificial intelligence that deals with the interaction between computers and human languages. NLP can be used for stock price forecasting and analysis by analyzing textual data sources, such as news articles, social media posts, or online reviews, and using them to make predictions based on various factors, such as sentiment, tone, opinion, etc.
- Support vector machines: Support vector machines (SVMs) are a type of machine learning algorithm that can classify or regress data based on a hyperplane that separates the data into different categories. SVMs can be used for stock price forecasting and analysis by finding the optimal hyperplane that can best fit the historical price data and using it to make predictions based on new data.
AI-powered stock price forecasting and analysis can help traders make better trading decisions and performance by using AI algorithms to analyze historical market and stock data, get investment ideas, build portfolios and automatically buy and sell stocks. AI-powered stock price forecasting and analysis can offer several benefits to traders, such as speed, accuracy, efficiency, scalability, and profitability.
The Role of Sentiment Analysis in AI-Driven Stock Trading
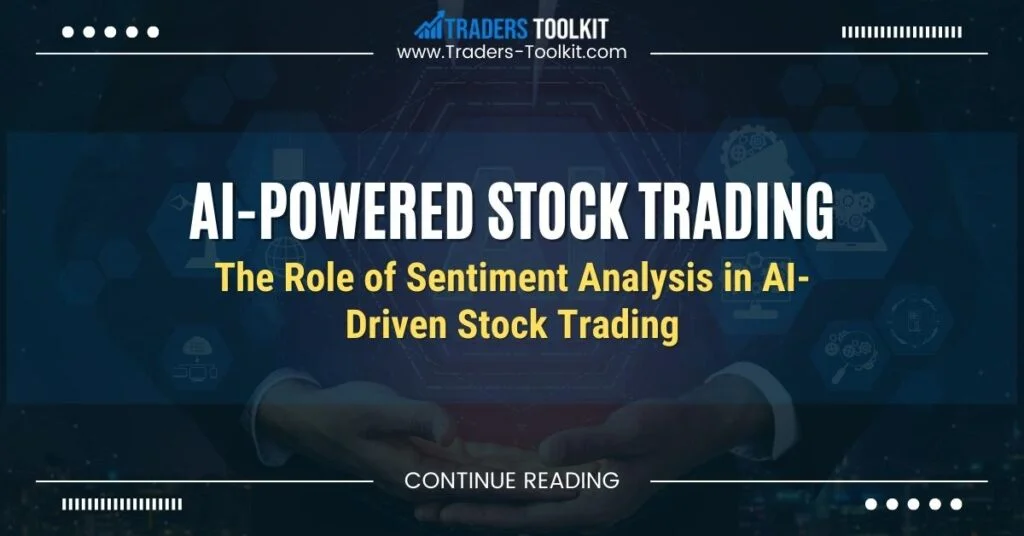
Another useful application of AI-powered stock trading is sentiment analysis. Sentiment analysis is the process of extracting and measuring the emotional tone or attitude of a text, such as positive, negative, or neutral. Sentiment analysis can be used in stock trading to gauge market sentiment and make informed investment choices.
Market sentiment is the overall feeling or mood of the market participants, such as investors, traders, analysts, etc., towards a particular stock, sector, or market. Market sentiment can affect the supply and demand of stocks and influence their price movements. For example, if the market sentiment is bullish, meaning that the market participants are optimistic and confident, the demand for stocks may increase and push their prices up. Conversely, if the market sentiment is bearish, meaning that the market participants are pessimistic and fearful, the supply of stocks may increase and pull their prices down.
Traditionally, market sentiment is measured by using indicators such as price trends, trading volume, volatility, etc. However, these indicators can be lagging or misleading, as they reflect the past or present actions of the market participants, not their future intentions or expectations. Moreover, these indicators can be influenced by external factors, such as news events, political developments, economic conditions, etc., that may not be directly related to the intrinsic value of a stock.
This is where sentiment analysis comes in. Sentiment analysis can measure market sentiment by analyzing textual data sources, such as news articles, social media posts, online reviews, etc., that express the opinions, feelings, or emotions of the market participants towards a particular stock, sector, or market. Sentiment analysis can offer several advantages over traditional indicators, such as:
- Timeliness: Sentiment analysis can provide real-time insights into the market sentiment and capture the changes or trends in the market mood.
- Relevance: Sentiment analysis can focus on the relevant data sources that are related to a specific stock, sector, or market and filter out the noise or irrelevant information.
- Accuracy: Sentiment analysis can provide more accurate and consistent results by using natural language processing (NLP) techniques to understand the meaning and context of the text.
- Actionability: Sentiment analysis can provide actionable recommendations or alerts based on market sentiment and help traders make better trading decisions.
Some examples of sources and methods of sentiment analysis are:
- News articles: News articles are one of the most common sources of sentiment analysis, as they provide factual and timely information about a stock, sector, or market. Sentiment analysis can use NLP techniques to extract keywords, topics, entities, etc., from news articles and assign them a sentiment score based on their polarity (positive or negative) and intensity (strong or weak).
- Social media posts: Social media posts are another popular source of sentiment analysis, as they reflect the opinions and emotions of millions of users who share their views on a stock, sector, or market. Sentiment analysis can use NLP techniques to classify social media posts into positive, negative, or neutral categories based on their language and tone.
- Online reviews: Online reviews are another useful source of sentiment analysis, as they provide feedback and ratings from customers who have used a product or service related to a stock, sector, or market. Sentiment analysis can use NLP techniques to analyze online reviews and extract aspects, features, or attributes that are important for customers and assign them a sentiment score based on their satisfaction or dissatisfaction.
Sentiment analysis can complement other forms of analysis and enhance trading strategies by providing traders with valuable insights into the market sentiment and helping them make informed investment choices.
AI vs. Human Traders: A Comparative Analysis of Performance

One of the most interesting questions that arises from AI-powered stock trading is how AI compares to human traders in terms of performance. Performance can be measured by various criteria, such as returns, risk-adjusted returns, drawdowns, volatility, etc. Performance can also be affected by various factors, such as speed, accuracy, emotion, bias, etc.
To answer this question, we need to compare the strengths and weaknesses of AI and human traders based on these criteria and factors. Here is a summary of some of the main points:
- Speed: AI has an advantage over human traders in terms of speed, as it can process huge amounts of data faster than humans and execute trades in milliseconds or microseconds. Human traders may be slower than AI due to physical limitations or cognitive constraints.
- Accuracy: AI has an advantage over human traders in terms of accuracy, as it can eliminate human errors and biases and provide more reliable and consistent results. Human traders may be less accurate than AI due to mistakes, miscalculations, or misjudgments.
- Efficiency: AI has an advantage over human traders in terms of efficiency, as it can optimize trading strategies and outcomes by finding the best opportunities and prices in the market. Human traders may be less efficient than AI due to suboptimal decisions or missed opportunities.
- Scalability: AI has an advantage over human traders in terms of scalability, as it can handle multiple trades and portfolios simultaneously and adapt to changing market conditions. Human traders may be less scalable than AI due to limited resources or capacity.
- Profitability: AI has an advantage over human traders in terms of profitability, as it can enhance trading performance and generate higher returns for traders. Human traders may be less profitable than AI due to lower speed, accuracy, efficiency, or scalability.
However, AI is not perfect and has some limitations and challenges that human traders may not have, such as:
- Transparency: AI has a disadvantage over human traders in terms of transparency, as it can be complex and opaque, making it difficult to understand how it works and why it makes certain decisions. Human traders may be more transparent than AI due to their ability to explain their rationale or logic.
- Accountability: AI has a disadvantage over human traders in terms of accountability, as it can make mistakes or cause harm, raising questions about who is responsible for its actions and outcomes. Human traders may be more accountable than AI due to their ability to take responsibility or liability.
- Privacy: AI has a disadvantage over human traders in terms of privacy, as it can collect and use personal or sensitive data from traders or consumers, raising concerns about data protection and security. Human traders may be more respectful of privacy than AI due to their ability to follow ethical or legal norms.
- Ethics: AI has a disadvantage over human traders in terms of ethics, as it can have social or environmental impacts, raising issues about fairness, justice, and sustainability. Human traders may be more ethical than AI due to their ability to follow moral or social values.
Therefore, the comparison between AI and human traders is not straightforward and depends on various criteria and factors. There is no definitive answer to which one is better or worse. The best approach may be to combine the strengths of both and avoid the weaknesses of both. For example, human traders can use AI as a tool or assistant to enhance their trading performance and results. Alternatively, AI can use human feedback or guidance to improve its trading performance and results.
AI and Risk Management: Safeguarding Investments in Volatile Markets

Another essential application of AI-powered stock trading is risk management. Risk management is the process of identifying, measuring, monitoring, and controlling the potential losses or uncertainties that may arise from trading activities. Risk management is vital for traders who want to safeguard their investments in volatile markets.
Volatility is the degree of variation or fluctuation in the price movements of stocks or markets over time. Volatility can be caused by various factors, such as supply and demand, news events, political developments, economic conditions, etc. Volatility can affect the profitability and stability of trading activities. For example, high volatility can create opportunities for higher returns but also expose traders to higher risks. Conversely, low volatility can reduce the risks but also limit the returns.
Traditionally, risk management relies on various tools and methods, such as portfolio diversification, asset allocation, hedging, stop-loss orders, etc., to reduce the exposure or impact of volatility on trading activities. However, these tools and methods have some limitations, such as:
- Data limitations: Risk management tools and methods require a lot of data to be accurate and reliable. However, not all data is available or accessible for all stocks or markets. Some data may also be outdated, incomplete or inaccurate.
- Human limitations: Risk management tools and methods involve a lot of human judgment and interpretation. However, humans can be prone to errors, biases or emotions that can affect their risk assessment and mitigation.
- Complexity limitations: Risk management tools and methods can be complex and time-consuming. They can also be difficult to apply to volatile or unpredictable markets or situations.
This is where AI comes in. AI-powered risk management uses machine learning algorithms to analyze market volatility and implement risk mitigation strategies based on various factors. AI-powered risk management can offer several advantages over traditional tools and methods, such as:
- Data advantages: AI can process huge amounts of data faster and more efficiently than humans. It can also access and use different types of data sources that may not be considered by traditional tools and methods.
- Human advantages: AI can eliminate human errors and biases and provide more objective and consistent results. It can also adapt to changing market conditions and learn from new data or feedback.
- Complexity advantages: AI can handle complex and nonlinear relationships between variables that may not be captured by traditional tools and methods. It can also provide more accurate and precise risk measures and controls for short-term or long-term horizons.
Some examples of AI tools and methods used for risk management are:
- Portfolio optimization: Portfolio optimization is the process of finding the optimal combination of assets that maximizes the expected return for a given level of risk or minimizes the risk for a given level of return. Portfolio optimization can use machine learning algorithms to analyze historical price data, correlations between assets, market trends, etc., and generate optimal portfolios based on various objectives and constraints.
- Hedging: Hedging is the process of reducing the exposure or impact of adverse price movements by taking an opposite position in a related asset or market. Hedging can use machine learning algorithms to identify and quantify the sources and levels of risk, such as market risk, currency risk, interest rate risk, etc., and determine the optimal hedging strategy and instruments, such as futures, options, swaps, etc.
- Stop-loss orders: Stop-loss orders are orders that automatically sell a stock when it reaches a predetermined price level to limit the losses or protect the profits. Stop-loss orders can use machine learning algorithms to dynamically adjust the stop-loss price based on various factors, such as volatility, momentum, trend, etc., and optimize the trade-off between risk and return.
AI-powered risk management can help traders reduce losses and increase returns in volatile markets by using machine learning algorithms to analyze market volatility and implement risk mitigation strategies based on various factors.
The Ethical Dilemmas of AI in Stock Trading: Opportunities and Concerns

Another important aspect of AI-powered stock trading is ethics. Ethics is the branch of philosophy that deals with the moral principles and values that guide human behavior and actions. Ethics can be applied to various domains and industries, such as healthcare, education, entertainment, and finance. In particular, ethics can be applied to stock trading, which is the activity of buying and selling stocks or shares of companies on the stock market.
Stock trading can have various ethical implications for traders, regulators, consumers, and society at large. For example, stock trading can affect the allocation of resources, the distribution of wealth, the creation of value, the protection of rights, etc. Stock trading can also involve various ethical issues and dilemmas, such as insider trading, market manipulation, discrimination, etc.
AI-powered stock trading can have both positive and negative ethical impacts on stock trading. On one hand, AI-powered stock trading can offer several opportunities for ethical improvement, such as:
- Transparency: AI-powered stock trading can increase transparency in the stock market by providing more information and insights to traders and regulators. For example, AI can help traders track and report their trades and portfolios. AI can also help regulators monitor and audit market activities and enforce compliance with rules and regulations.
- Accountability: AI-powered stock trading can increase accountability in the stock market by providing more evidence and justification for trading decisions and outcomes. For example, AI can help traders explain the rationale or logic behind their trades. AI can also help regulators identify and investigate any suspicious or fraudulent behavior in the market.
- Privacy: AI-powered stock trading can increase privacy in the stock market by providing more security and control over personal or sensitive data. For example, AI can help traders encrypt or anonymize their data before sharing or storing it. AI can also help regulators protect or restrict access to confidential or proprietary data in the market.
- Ethics: AI-powered stock trading can increase ethics in the stock market by providing more guidance and support for ethical decision-making. For example, AI can help traders follow ethical principles and values in their trading activities. AI can also help regulators establish and enforce ethical standards and norms in the market.
On the other hand, AI-powered stock trading can also pose several concerns for ethical deterioration, such as:
- Transparency: AI-powered stock trading can decrease transparency in the stock market by creating more complexity and opacity in trading activities. For example, AI algorithms can be difficult to understand or interpret by traders or regulators. AI algorithms can also be manipulated or hacked by malicious actors in the market.
- Accountability: AI-powered stock trading can decrease accountability in the stock market by creating more uncertainty and ambiguity in trading responsibilities and consequences. For example, AI algorithms can make mistakes or cause harm that may not be anticipated or intended by traders or regulators. AI algorithms can also shift or evade liability or blame by traders or regulators.
- Privacy: AI-powered stock trading can decrease privacy in the stock market by creating more vulnerability and exposure to personal or sensitive data. For example, AI algorithms can collect or use data without consent or awareness by traders or consumers. AI algorithms can also leak or disclose data to unauthorized or untrustworthy parties in the market.
- Ethics: AI-powered stock trading can decrease ethics in the stock market by creating more conflicts and challenges for ethical values and principles. For example, AI algorithms may not align with human morals or social norms in their trading activities. AI algorithms may also undermine human dignity or autonomy by traders or consumers.
Therefore, AI-powered stock trading has both opportunities and concerns for ethics in stock trading. There is no definitive answer to whether AI is good or bad for ethics in stock trading. The best approach may be to balance the benefits and risks of AI and ensure that AI is used in a responsible and ethical manner. For example, traders and regulators can adopt ethical frameworks and guidelines to govern the development and use of AI in stock trading. Alternatively, traders and regulators can involve human oversight or participation in the design and implementation of AI in stock trading.
AI-Powered Portfolio Management: Creating Diversified and Profitable Portfolios

Another valuable application of AI-powered stock trading is portfolio management. Portfolio management is the process of selecting and managing a collection of assets that meets the investment objectives and constraints of a trader. Portfolio management is important for traders who want to create diversified and profitable portfolios for long-term success.
Diversification is the strategy of investing in different types of assets that have low or negative correlations with each other. Diversification can reduce the overall risk and volatility of a portfolio by spreading the exposure across different sources of returns. Profitability is the measure of the financial performance and results of a portfolio over time. Profitability can be influenced by various factors, such as returns, costs, fees, taxes, etc.
Traditionally, portfolio management relies on various tools and methods, such as asset allocation, rebalancing, performance measurement, etc., to create and manage diversified and profitable portfolios. However, these tools and methods have some limitations, such as:
- Data limitations: Portfolio management tools and methods require a lot of data to be accurate and reliable. However, not all data is available or accessible for all assets or markets. Some data may also be outdated, incomplete, or inaccurate.
- Human limitations: Portfolio management tools and methods involve a lot of human judgment and interpretation. However, humans can be prone to errors, biases, or emotions that can affect their portfolio selection and management.
- Complexity limitations: Portfolio management tools and methods can be complex and time-consuming. They can also be difficult to apply to dynamic or uncertain markets or situations.
This is where AI comes in. AI-powered portfolio management uses machine learning algorithms to analyze historical price data, correlations between assets, market trends, etc., and generate optimal portfolios based on various objectives and constraints. AI-powered portfolio management can offer several advantages over traditional tools and methods, such as:
- Data advantages: AI can process huge amounts of data faster and more efficiently than humans. It can also access and use different types of data sources that may not be considered by traditional tools and methods.
- Human advantages: AI can eliminate human errors and biases and provide more objective and consistent results. It can also adapt to changing market conditions and learn from new data or feedback.
- Complexity advantages: AI can handle complex and nonlinear relationships between variables that may not be captured by traditional tools and methods. It can also provide more accurate and precise portfolio measures and controls for short-term or long-term horizons.
Some examples of AI models and techniques used for portfolio management are:
- Reinforcement learning: Reinforcement learning is a type of machine learning algorithm that learns from its own actions and experiences by interacting with an environment. Reinforcement learning can be used for portfolio management by learning from historical price data, rewards (returns), and penalties (risks) and finding the optimal portfolio policy (allocation) that maximizes the expected return for a given level of risk or minimizes the risk for a given level of return.
- Genetic algorithms: Genetic algorithms are a type of machine learning algorithm that mimics the process of natural selection and evolution. Genetic algorithms can be used for portfolio management by generating a population of portfolios (solutions) based on various criteria (genes), such as returns, risks, costs, etc., and selecting the best portfolios (survivors) based on their fitness (performance) over multiple generations (iterations).
- Multi-objective optimization: Multi-objective optimization is a type of machine learning algorithm that solves problems that have more than one objective or goal. Multi-objective optimization can be used for portfolio management by finding the optimal trade-off between multiple objectives or constraints, such as returns, risks, costs, etc., using various methods, such as Pareto optimality, weighted sum method, etc.
AI-powered portfolio management can help traders achieve their financial goals and objectives by using machine learning algorithms to analyze historical market and stock data, get investment ideas, build portfolios, and automatically buy and sell stocks. AI-powered portfolio management can offer several benefits to traders, such as diversification, profitability, stability, and adaptability.
AI’s Impact on Technical Analysis: Enhancing Trading Strategies
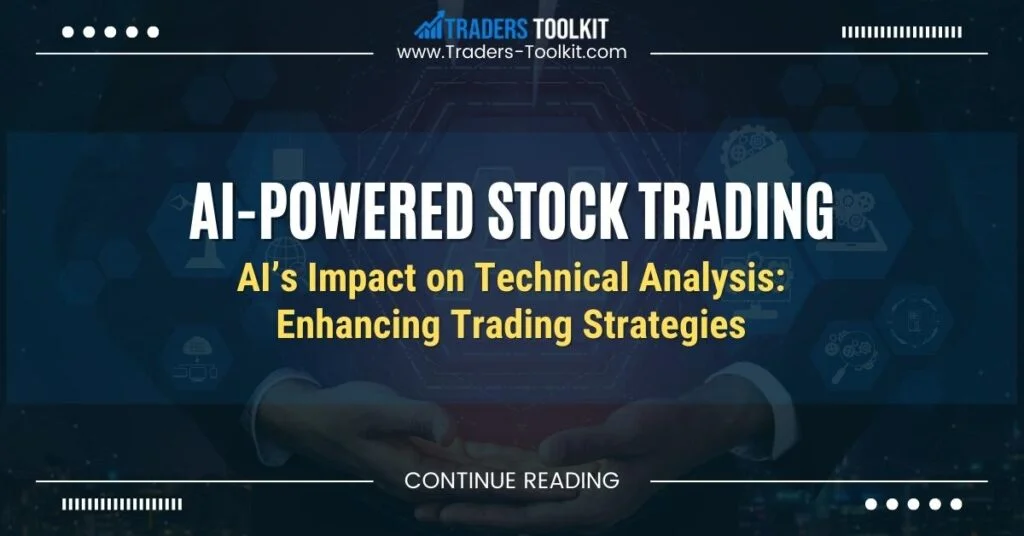
Another beneficial application of AI-powered stock trading is technical analysis. Technical analysis is the process of studying the price patterns, trends, indicators, etc., of a stock or market using statistical tools and charts. Technical analysis can be used in stock trading to enhance trading strategies and outcomes.
Trading strategies are the plans or methods that traders use to buy and sell stocks or shares of companies on the stock market. Trading strategies can be based on various criteria, such as time horizon, risk appetite, trading style, etc. Trading outcomes are the results or consequences of trading activities, such as returns, risks, costs, etc.
Traditionally, technical analysis relies on various tools and methods, such as trend lines, moving averages, oscillators, etc., to enhance trading strategies and outcomes. However, these tools and methods have some limitations, such as:
- Data limitations: Technical analysis tools and methods require a lot of data to be accurate and reliable. However, not all data is available or accessible for all stocks or markets. Some data may also be outdated, incomplete, or inaccurate.
- Human limitations: Technical analysis tools and methods involve a lot of human judgment and interpretation. However, humans can be prone to errors, biases, or emotions that can affect their technical analysis and trading decisions.
- Complexity limitations: Technical analysis tools and methods can be complex and time-consuming. They can also be difficult to apply to dynamic or uncertain markets or situations.
This is where AI comes in. AI-powered technical analysis uses machine learning algorithms to automate pattern recognition and provide more accurate insights to traders. AI-powered technical analysis can offer several advantages over traditional tools and methods, such as:
- Data advantages: AI can process huge amounts of data faster and more efficiently than humans. It can also access and use different types of data sources that may not be considered by traditional tools and methods.
- Human advantages: AI can eliminate human errors and biases and provide more objective and consistent results. It can also adapt to changing market conditions and learn from new data or feedback.
- Complexity advantages: AI can handle complex and nonlinear relationships between variables that may not be captured by traditional tools and methods. It can also provide more accurate and precise technical measures and indicators for short-term or long-term horizons.
Some examples of AI models and techniques used for technical analysis are:
- Convolutional neural networks: Convolutional neural networks (CNNs) are a type of deep learning algorithm that uses multiple layers of filters to extract features from images or other types of data. CNNs can be used for technical analysis by learning from historical price charts and generating predictions based on various patterns, such as trends, breakouts, reversals, etc.
- Recurrent neural networks: Recurrent neural networks (RNNs) are a type of deep learning algorithm that uses feedback loops to process sequential or temporal data. RNNs can be used for technical analysis by learning from historical price sequences and generating predictions based on various indicators, such as momentum, volatility, etc.
- Support vector machines: Support vector machines (SVMs) are a type of machine learning algorithm that can classify or regress data based on a hyperplane that separates the data into different categories. SVMs can be used for technical analysis by finding the optimal hyperplane that can best fit the historical price data and using it to make predictions based on new data.
AI-powered technical analysis can help traders improve their trading strategies and outcomes by using machine learning algorithms to automate pattern recognition and provide more accurate insights to traders.
The Future of AI in Stock Trading: Trends and Predictions

The last section of this article will provide a forward-looking perspective on the evolving role of AI in the stock market and speculate on future developments that traders should watch for. AI-powered stock trading is not a static or fixed phenomenon but a dynamic and progressive one that is constantly changing and improving. Therefore, it is important for traders to keep up with the latest trends and predictions that are shaping the future of AI in stock trading.
Some of the trends and predictions that are shaping the future of AI in stock trading are:
- Big data: Big data refers to the large volumes of structured or unstructured data that are generated or collected from various sources at high speed and variety. Big data can provide more information and insights for AI-powered stock trading by enabling more comprehensive and granular analysis and prediction.
- Cloud computing: Cloud computing refers to the delivery of computing services over the internet, such as storage, processing, networking, etc. Cloud computing can provide more flexibility and scalability for AI-powered stock trading by enabling more access and use of AI tools and resources.
- Blockchain: Blockchain refers to a distributed ledger system that records and verifies transactions using cryptography and consensus mechanisms. Blockchain can provide more security and transparency for AI-powered stock trading by enabling more trust and verification of AI activities and outcomes.
- Quantum computing: Quantum computing refers to a new paradigm of computing that uses quantum mechanics to perform operations that are impossible or impractical for classical computers. Quantum computing can provide more speed and power for AI-powered stock trading by enabling more complex and efficient AI algorithms and models.
Some of the innovative applications and opportunities that may emerge from AI in stock trading are:
- Robo-advisors: Robo-advisors are online platforms or apps that provide automated financial advice or guidance to investors based on their goals, preferences, and risk profiles. Robo-advisors can use AI to analyze market data, generate investment recommendations, execute trades, and manage portfolios.
- Social trading platforms: Social trading platforms are online platforms or apps that allow investors to interact, share, and learn from each other. Social trading platforms can use AI to enhance social trading features, such as ranking, rating, following, copying, etc.
- Smart contracts: Smart contracts are self-executing contracts that are encoded on a blockchain and triggered by predefined conditions or events. Smart contracts can use AI to automate and enforce trading agreements, such as payments, deliveries, settlements, etc.
AI-powered stock trading is an exciting and promising field that is transforming the stock market landscape. Traders who want to take advantage of AI-powered stock trading should be aware of the benefits and challenges, the types, tools and techniques, the ethical implications, and the future developments that AI-powered stock trading entails. By doing so, traders can leverage AI technology to improve their trading performance and results.
Conclusion
This article has provided a comprehensive overview of AI-powered stock trading, including its impact on traders, regulators, consumers, and society at large. We have discussed some of the applications, benefits, and challenges of AI-powered stock trading, such as:
- Trading bots: Trading bots are software programs that use predefined rules or algorithms to automatically buy and sell stocks on behalf of traders. Trading bots can make stock trading more accessible, affordable, diverse, and profitable for retail investors.
- Stock price forecasting and analysis: Stock price forecasting and analysis is the process of predicting the future price movements of stocks based on historical data, current trends, and other factors. Stock price forecasting and analysis can make stock trading more accurate, efficient, scalable, and profitable for traders.
- Sentiment analysis: Sentiment analysis is the process of extracting and measuring the emotional tone or attitude of a text. Sentiment analysis can make stock trading more timely, relevant, accurate, and actionable for traders.
- Technical analysis: Technical analysis is the process of studying the price patterns, trends, indicators, etc., of a stock or market using statistical tools and charts. Technical analysis can make stock trading more automated, insightful, precise, and effective for traders.
- Risk management: Risk management is the process of identifying, measuring, monitoring, and controlling the potential losses or uncertainties that may arise from trading activities. Risk management can make stock trading more secure, stable, adaptable, and profitable for traders.
- Portfolio management: Portfolio management is the process of selecting and managing a collection of assets that meets the investment objectives and constraints of a trader. Portfolio management can make stock trading more diversified, profitable, stable, and adaptable for traders.
We have also discussed some of the ethical considerations surrounding AI-powered stock trading, such as:
- Transparency: Transparency is the degree of clarity or openness in trading activities. Transparency can be increased or decreased by AI-powered stock trading depending on the complexity and opacity of AI algorithms or the availability and quality of data.
- Accountability: Accountability is the degree of responsibility or liability for trading actions or outcomes. Accountability can be increased or decreased by AI-powered stock trading depending on the uncertainty and ambiguity of AI decisions or the identification and investigation of AI errors or harms.
- Privacy: Privacy is the degree of protection or control over personal or sensitive data. Privacy can be increased or decreased by AI-powered stock trading depending on the vulnerability and exposure of data collection or use by AI algorithms or the encryption or anonymization of data sharing or storage by AI algorithms.
- Ethics: Ethics is the degree of alignment or conflict with moral principles or values in trading activities. Ethics can be increased or decreased by AI-powered stock trading depending on the guidance and support for ethical decision-making by AI algorithms or the impact on human dignity or autonomy by AI algorithms.
Finally, we have discussed some of the future developments that traders should watch for in AI-powered stock trading, such as:
- Big data: Big data can provide more information and insights for AI-powered stock trading by enabling more comprehensive and granular analysis and prediction.
- Cloud computing: Cloud computing can provide more flexibility and scalability for AI-powered stock trading by enabling more access and use of AI tools and resources.
- Blockchain: Blockchain can provide more security and transparency for AI-powered stock trading by enabling more trust and verification of AI activities and outcomes.
- Quantum computing: Quantum computing can provide more speed and power for AI-powered stock trading by enabling more complex and efficient AI algorithms and models.
- Robo-advisors: Robo-advisors can provide more automated and personalized financial advice or guidance to investors based on their goals, preferences, and risk profiles.
- Social trading platforms: Social trading platforms can provide more interactive and collaborative trading experiences for investors by allowing them to interact, share, and learn from each other.
- Smart contracts: Smart contracts can provide more automated and enforceable trading agreements between parties by using blockchain technology.
AI-powered stock trading is an exciting and promising field that is transforming the stock market landscape. Traders who want to take advantage of AI-powered stock trading should be aware of the benefits and challenges, the types, tools, and techniques, the ethical implications, and the future developments that AI-powered stock trading entails. By doing so, traders can leverage AI technology to improve their trading performance and results.
We hope you enjoyed reading this article and learned something new about AI-powered stock trading. If you have any questions or feedback, please feel free to leave a comment below. Thank you for your attention and happy trading!